The full feature profile for Aylin can be found here
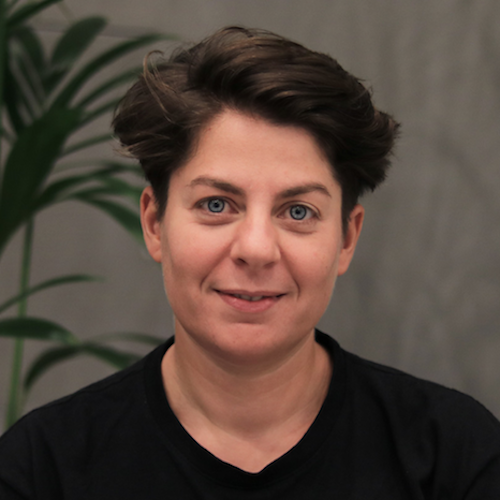
1. What were some of the benefits and challenges of making the transition from pure mathematics into bioinformatics? If you could go back in time, what advice would you give yourself while making that transition?
Transitioning was really fun but also quite hard - I didn’t understand the biology papers I was reading. Mostly, I really hated that there were SO MANY ambiguous definitions in biology! The benefits for me were mainly that I was finally able to work closely with several people. Research in pure maths can be quite lonely sometimes. And that you can plot some data - ‘examples’ in pure maths are still really theoretical!
2. Coming from a background in mathematics and combinatorics, do you feel like you have a different or unique perspective on your research areas than other bioinformaticians who come from different backgrounds?
My thought processes are definitely a bit different from my colleagues with different backgrounds - if I can represent something as a graph-theoretical problem I will even if it’s during a pub quiz :) And maybe I’m a bit more comfortable tweaking published methods a bit to suit my needs compared to people with a more biological background.
3. What has your experience of the gender balance in the general areas of mathematics and bioinformatics and your particular areas of machine learning and gene regulation been since you started your research career? Have you seen any significant changes since you started in these fields?
There are just not many women in mathematics and especially pure maths - and while most male mathematicians are lovely people, you can feel quite alien if you are ‘one of the few’. I used to wear fake glasses in seminars and conferences just to look a bit more geeky - back then I looked very girly. Going into biology definitely shifted that, all of the sudden I was surrounded by women (and had to queue for the women’s toilets, definitely a downside.. ). But there is still a problem with these women not getting into leadership roles, so when you look up your career ladder it still seems to be populated mainly by men. This is true in academia but also across tech companies. It’s frustrating to think that not much has changed since I started my PhD in 2008 except for some box-ticking exercises that don’t really change much (or even end up creating additional work for the few women, like having to conduct many more interviews to achieve gender balance on the panel). I think there has been a bit of change since the MeToo movement. At least gender balance in the workforce is now a subject that is more often discussed and people in charge do realise they have to actively address it to change the status quo. Sadly, I’m not sure I would call it significant, I’m still ‘one of the few’ when I look around.
4. Would you say there are particular assets or challenges to being a woman in bioinformatics?
Unfortunately, the challenges are still the good old ones that any woman faces when working in a male-dominated field: stereotypes mean you have to work twice as hard to be judged comparable to a guy, you’re always assumed to be more junior than the guy next to you and so on and so on. I think the best asset for a woman in bioinformatics are the other women. There is a certain camaraderie amongst us, we all have stories and experiences that reflect our individual set of intersecting minority backgrounds, whether that is gender, sexual orientation, or ethnicity. I think for a long time there was a feeling of if we just work hard enough and ‘lean in’ all will be good, so we didn’t even complain to each other. The fact that we can be more outspoken about the problems means also that we have started talking to each other about it and can help each other out.
5. Having made the transition between from an academic research lab, and industry-based research, what are the biggest differences you've noticed between academia and industry research environments? Do you have any advice for young researchers making a similar transition?
For me the transition has been a really positive experience that has surprised me a lot. As an academic I viewed “the industry” as this dead place where you’re paid to do your job, that you work ‘for the man’, without being innovative or creative, and I worried a lot that it would diminish the excitement I have for my work. Instead what I’ve found (at least at BenevolentAI) is an incredibly collaborative environment where your opinions count a lot and your innovative ideas are encouraged for you to try them out. I was amazed how quickly someone would drop what they were doing to help you out and how quickly a comment like ‘we should try this’ can become a quarterly initiative for your team if it’s a good idea. My advice would be to try and network and just talk to people that work at the kind of companies you are considering. This could be at meetups for example or through other networks. For example the https://twitter.com/AIClubGenderMin is an amazing group of people that will answer questions. If it’s a well written email or message to someone that shows you have researched the person should give you a reply as well (especially if you can praise a detail in their latest publication ;) ). This seems to be something that guys don’t have any problem doing but somehow women shy away from (judging on my own LinkedIn inbox and my own experience). Another way is trying it out in an internship - we have just published the summer internships at BenevolentAI and I have a project around deep learning in genomics in the mix, so if you’re interested please apply https://www.benevolent.com/internships, deadline is 12th of February!
6. What future areas and avenues of research are you most excited about?
I’m really excited about what’s to come in terms of machine learning in genomics and how we will use it to improve people’s lives through new drugs or better disease management. I think we are only at the start of seeing what is possible, the application of for example deep learning in genomics is fairly new and seems to be trailing behind a bit - often enough we tweak or apply models that were developed for other problems and domains. In addition, new data sources and modalities are coming out almost every day and soon we will have massive cohorts of patient-derived molecular data together with their clinical features. I can’t wait to see how we will integrate all these different data with machine learning and previous knowledge to really understand the complexity of gene regulation in particular in a disease context.
7. Could you also describe what led you to start CrAIC and what your experience was with that?
When I started using more machine learning in my research, I searched for people that had more experience than me to ask some questions and get some help. It turned out that there were a few people at the institute in the same boat as me and we first teamed up, shared contacts and tried to solve our problems over coffee. I then thought, why not do this with all the people we know that have more expertise than us and we offer them a beer in return. So the first AI club happened in the Francis Crick Institute with internal researchers as well as our friends/mentors from BenevolentAI and UCL. During that meeting we decided it would be good to have a seminar to give the meetings more structure and us more reason to enjoy our beers and discussions afterwards. And so the Crick AI club was born https://twitter.com/CrickAIClub. For me it was always really important to emphasise the informality of the seminars (and subsequent discussions) and to have the networking drinks afterwards as the main aim was to connect like-minded people across London. The organisation was a little challenging in the beginning, but I soon got the hang of booking the rooms, the drinks and making sure external guests could sign in. I’ve made some amazing connections through it and I have learned so much from the speakers and the erupting discussions and questions. I’m really grateful to everyone who has helped out, given a talk or just turned up!
Comments